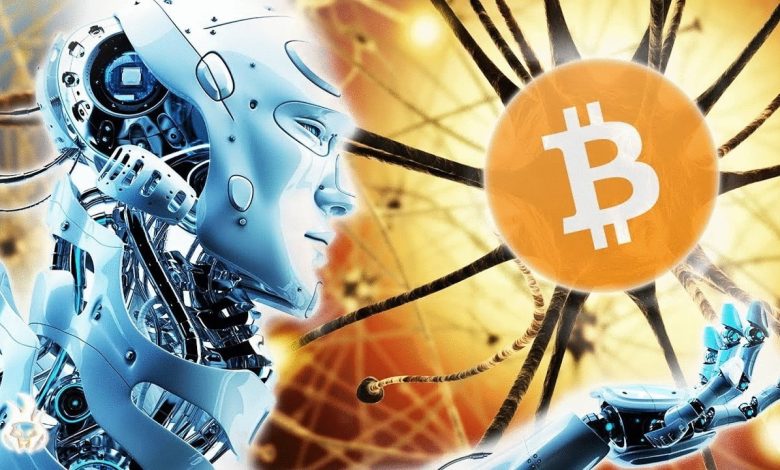
The last two weeks have been an absolute whirlwind in the world of generative artificial intelligence (AI), with groundbreaking new releases and cutting-edge integrations taking place. OpenAI released its highly anticipated GPT-4 model, Midjourney unveiled its latest V5 model, and Stanford released their Alpaca 7B language model. Meanwhile, Google launched generative AI across its entire Workspace suite, while Anthropic introduced its AI assistant Claude, and Microsoft integrated its powerful generative AI tool Copilot into the Microsoft 365 suite.
The pace of AI development and adoption is showing no signs of slowing down as businesses have begun to realize the value of AI and automation and the need to adopt these technologies to remain competitive in the market.
Despite the seemingly smooth progress of AI development, there are underlying challenges and bottlenecks that must be addressed. As more businesses and consumers embrace AI, a bottleneck is emerging around computing power. The computations required for AI systems is doubling every few months, while the supply of computing resources struggles to keep pace. Furthermore, the cost of training large-scale AI models continues to soar, with an increase of approximately 3100% per year over the past decade.
This trend towards rising costs and increasing resource demands needed to develop and train cutting-edge AI systems is resulting in centralization, where only entities with massive budgets are able to conduct research and produce models. However, several crypto-based projects are building decentralized solutions to address these issues using open compute and machine intelligence networks.
Primer on AI and ML
The advancement of AI is driven by three main factors:
- Algorithmic innovation: Researchers are constantly developing new algorithms and techniques that allow AI models to process and analyze data more efficiently and accurately.
- Data: AI models rely on large datasets as the fuel for their training, enabling them to learn from patterns and relationships within the data.
- Compute: The complex calculations necessary for training AI models require large amounts of computational processing power.
However, there are two main problems that are hindering the development of AI. Back in 2021, obtaining data was the top challenge facing AI companies in their pursuit of AI development. Last year, compute-related issues surpassed data as a challenge, specifically due to the lack of on-demand access to compute resources, driven by high levels of demand.
The second problem is related to inefficiencies in algorithmic innovation. While researchers continue to make incremental improvements to models by building on previous ones, the intelligence, or patterns, extracted by these models is always lost.
Let’s dig deeper into these problems.
Compute Bottleneck and Decentralized AI
Training foundational machine learning models requires extensive resources, often involving the use of large numbers of GPUs over extended periods of time. For instance, Stablility.AI needed 4,000 Nvidia A100 GPUs running in AWS’ cloud to train their AI models, which cost them more than $50 million in a month. OpenAI’s GPT-3, on the other hand, was trained using 1,000 Nvidia V100 GPUs, costing $12 million.
AI companies are often faced with two choices: investing in their own hardware and sacrificing scalability, or opting for cloud providers and paying inflated prices. While larger companies can afford to choose the latter, smaller companies may not have that luxury. With the rising cost of capital, startups are being forced to cut back on cloud spending, even as the cost of expanding infrastructure for large cloud providers remains largely unchanged.
The high cost of computing for AI creates significant obstacles for researchers and organizations pursuing advancements in the field. Currently, there is a pressing need for an affordable, on-demand serverless compute platform for ML work, which is absent in the traditional computing landscape. Fortunately, several crypto projects are working to develop decentralized machine learning compute networks that can address this need.
Blockchain and machine learning
Deep learning has gained huge traction in recent years because of its potential to make informed decisions. A large portion of today’s deep learning systems are based on centralized servers and fall short in providing operational transparency, traceability, reliability, security, and trusted data provenance features. Also, training deep learning models by utilizing centralized data is vulnerable to the single point of failure problem. In this paper, we explore the importance of integrating blockchain technology with deep learning. We review the existing literature focused on the integration of blockchain with deep learning. We classify and categorize the literature by devising a thematic taxonomy based on seven parameters; namely, blockchain type, deep learning models, deep learning specific consensus protocols, application area, services, data types, and deployment goals. We provide insightful discussions on the state-of-the-art blockchain-based deep learning frameworks by highlighting their strengths and weaknesses. Furthermore, we compare the existing blockchain-based deep learning frameworks based on four parameters such as blockchain type, consensus protocol, deep learning method, and dataset. Finally, we present important research challenges which need to be addressed to develop highly trustworthy deep learning frameworks.
Blockchain technology enables establishing the provenance of machine learning models, thus leading to trusted Artificial intelligence (AI) systems. Blockchain technology presents a robust system and can incentivize the participants who share their data (data trading) which is used to train machine learning models.